Authors: Rajesh Singla and Revathidevi Somasundaram
Imagine a tool with the ability to handle large amounts of data, uncover concealed patterns independently, and create content based on the knowledge it has acquired. This is the potential of generative AI, which is powered by a technology known as Large Language Models (LLMs).
Generative AI, using LLMs, is revolutionizing artificial intelligence by effortlessly processing and producing content that resembles human language. An LLM can be likened to an exceptionally intelligent translator who not only understands language syntax and structure but also the background and nuances of words. It utilizes complex calculations to analyze vast amounts of text, determining the likelihood of different words co-occurring and constructing sentences through intricate algorithms.
This is where things get interesting: LLMs (such as GPT-4, Gemini, or LLaMa) use robust artificial neural networks designed to imitate the human brain. These machines can understand relationships between words and phrases by breaking sentences into smaller parts and converting them into vectors. This enables the LLM to understand your information and how it all fits together.
Nevertheless, data can restrict LLMs despite their power, leading to old or partial information. Retrieval-augmented generation (RAG) is a solution to this problem. RAG combines data retrieval from vast knowledge bases with AI-driven content creation to provide accurate outputs. This means that RAG guarantees better productivity in terms of speed and scalability while ensuring diversity in the solutions offered for different contexts by keeping everything within one system.
In today’s era, when businesses are looking to make use of AI innovations, it becomes crucial to understand how RAG works and becomes critical for fully utilizing the potential, which can further enhance operations and pave a long way to improve customer interactions.  This blog focuses majorly on Retrieval Augmented Generation:
Generative AI Application: Retrieval Augmented Generation (RAG)
While LLMs have excellent capabilities, they also have their limitations. They heavily rely on the data they were trained on, which might give outdated or incomplete information, particularly in rapidly changing areas. This can be fixed by combining LLMs with Retrieval-Augmented Generation (RAG). In RAG, a model retrieves relevant knowledge from a vast dataset or knowledge base before utilizing it to generate a response, making sure that what comes out is sensible and accurate.
Imagine having a powerful tool that can:
- Gather the right information: Consider it your personalized research assistant, searching through enormous databases (data comes in different forms from documents) to find exactly what you need.
- Write clear and compelling content: RAG translates that data into well-written pieces that are audience-specific and meet all the requirements.
That’s the wonder of RAG (Retrieval-Augmented Generation). It brings together two approaches:
- Data Retrieval—Like an assistant or librarian, RAG looks for helpful information relevant to your task from a large dataset (such as industry reports, customer reviews, or social media data).
- Content Generation – Once it has found the relevant data, like the one the user asked for, RAG utilizes the filtered data to create concise, easy-to-understand pieces that capture attention. To have a glimpse, you can think of product descriptions, marketing texts, personalized emails, etc. – all of which have been generated automatically.
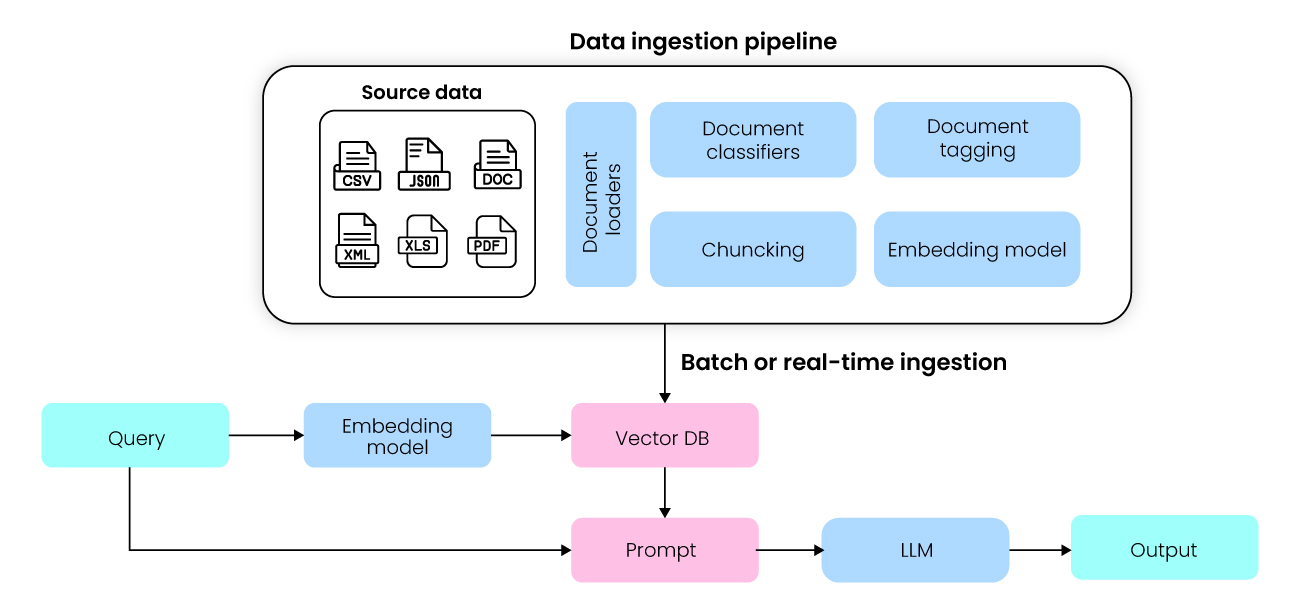
Figure 1: High-level architecture for RAG
RAG can utilize the following datasets:
- Marketing & Sales: Competitor analysis data, customer reviews, and market research reports.
- Finance: Financial news articles, company filings, etc.
- Human Resources: Training materials, employee feedback surveys, and job descriptions.
- Life Science: Personalized health recommendations and up-to-date medical information; collate and retrieve patient information, clinical trial data, expert opinions, research papers, etc.
Benefits of Generative AI (RAG):
- Precision: The system is based on provided data, reducing the risk of inaccuracy or obsolescence.
- Saving time and resources by automating content creation tasks.
- Flexibility: RAG can work with a large amount of data and adapt it to different content requirements.
- Uniformity: Keep brand voice consistent throughout all content you produce.
Conclusion:
Retrieval-augmented generation combines the exactness inherent in retrieval-based systems with the versatility of generative AI. In other words, organizations should deliver quick, accurate, and personalized assistance to users, eventually increasing their satisfaction and loyalty. As Artificial Intelligence technology advances, we may anticipate RAG playing an ever more critical part in ensuring outstanding customer experiences.